How Generative AI Helps with Fraud Prevention in Healthcare
Healthcare fraud is a pervasive problem that costs individuals and insurance companies billions of dollars each year. According to the National Health Care Anti-Fraud Association, over $54 billion is lost annually due to fraudulent and illegal medical charges. As the volume and complexity of healthcare data continue to grow, traditional fraud detection methods are struggling to keep pace. This is where generative AI-powered fraud detection can make a significant difference. In this blog, we will discuss five ways in which generative AI helps with fraud detection in healthcare.
Applications of Gen AI in preventing healthcare fraud
1. Automated Detection of Unusual Billing Patterns
Generative AI in healthcare can analyze large datasets to spot unusual billing patterns that may indicate fraudulent activities. By continuously learning from historical data, AI models can identify discrepancies such as overbilling, upcoding, or billing for never-rendered services. Here’s an example to help you understand better.
Imagine a hospital network with multiple clinics. AI algorithms monitor all claims submitted by healthcare providers. One day, the system flags a pattern where a particular clinic submits a significantly higher number of outpatient procedures than similar clinics in the region.
The AI detects a sudden spike in claims for a specific procedure that historically is rarely done. It alerts the fraud detection team to investigate. Upon review, it was found that a small group of doctors was overbilling for unnecessary procedures, which could have otherwise gone unnoticed.
This benefit of generative AI helps healthcare organizations prevent overbilling and ensure that claims are legitimate, saving money and maintaining compliance with healthcare regulations.
2. Predictive Analytics for Identifying High-Risk Providers
Generative AI leverages predictive analytics to assess which healthcare providers or organizations may pose a higher risk for fraudulent activities. Using historical data on fraud trends, AI systems can predict which providers are more likely to engage in dishonest behaviors and flag them for further scrutiny.
Here’s a simple example to explain the predictive analytics benefit of Gen AI.
A health insurance company uses AI to monitor claims across different medical facilities. The AI identifies a hospital with an unusually high rate of denied claims for expensive treatments. Based on historical data, the AI identifies similar trends in the past and flags the facility as a potential risk for fraudulent claims.
Upon deeper analysis, the fraud team uncovers a scheme where certain physicians were billing for treatments that were not supported by patient records. The predictive AI model, in this case, helped the insurance company take preemptive action, stopping the fraudulent activity before it could escalate.
By proactively identifying high-risk providers, generative AI empowers healthcare institutions to reduce their exposure to fraud.
3. Enhanced Claims Review with Natural Language Processing
Generative AI equipped with Natural Language Processing can analyze clinical notes, medical records, and claims data in real-time. NLP algorithms help identify inconsistencies between what is documented and what is billed. This allows AI systems to detect anomalies more accurately and efficiently compared to manual review.
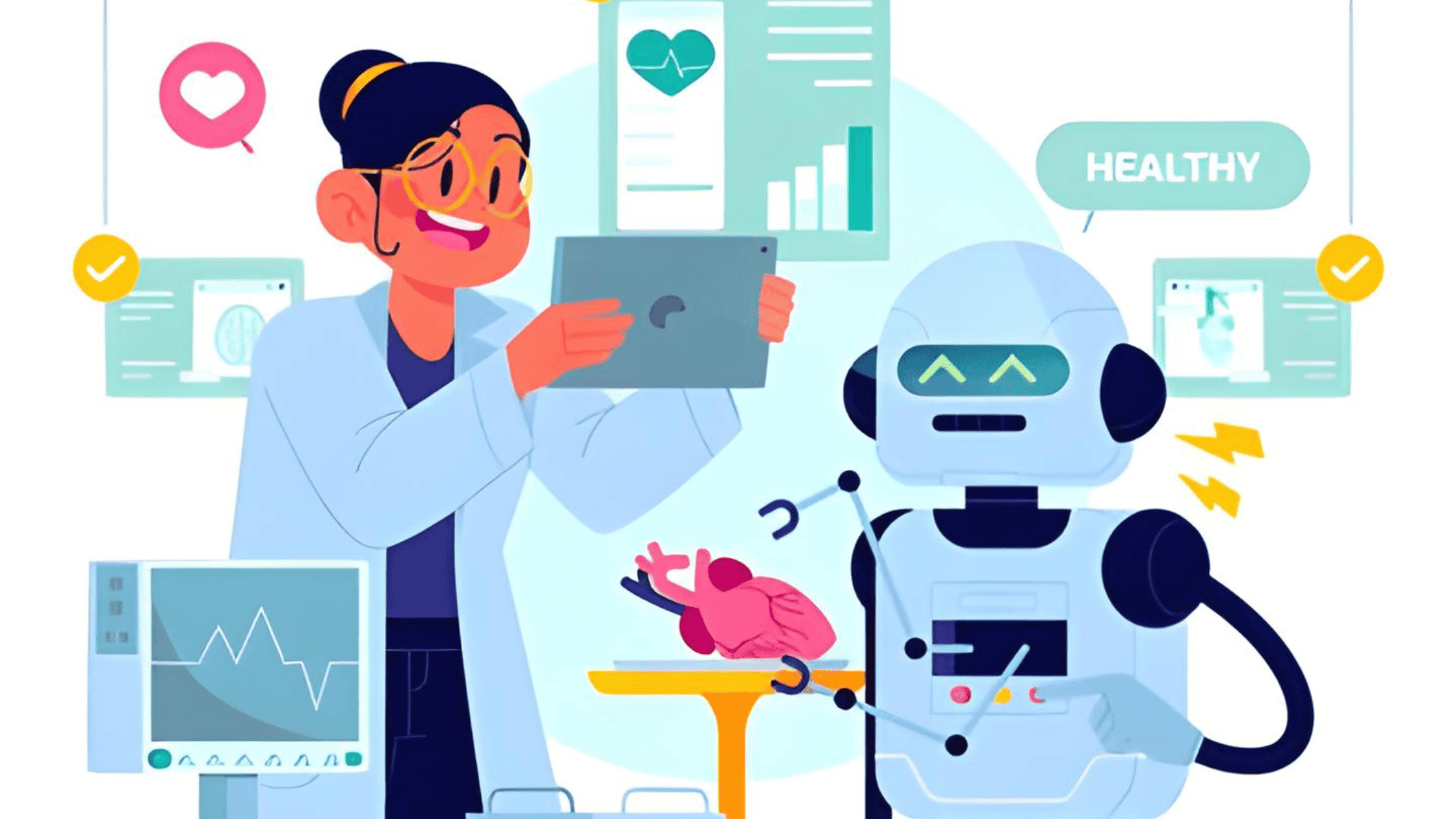
Consider a healthcare provider that processes hundreds of thousands of claims every month.
Traditionally, claims adjusters would manually review these claims, but it would take considerable time, often leading to human error. With generative AI using NLP, the system scans medical records and cross-references them with the claims submitted. In one case, the AI flags a claim where the patient’s record shows a minor consultation, but the claim includes charges for an intensive diagnostic procedure. The AI alerts the provider’s fraud team, who then verify that the procedure was never conducted, thus preventing an incorrect payment.
NLP-enabled AI systems improve the accuracy of claims auditing, reduce false positives, and expedite the detection of fraudulent claims.
4. Anomaly Detection in Patient and Provider Behavior
Generative AI can monitor both patient and provider behavior over time to detect anomalies that may indicate fraudulent activities. By understanding typical patterns of behavior, AI can pinpoint when deviations occur that might be indicative of fraud, such as a sudden surge in a provider’s services or abnormal patient interactions.
Let’s say a hospital system uses AI to track patient visits and the services they receive. The AI system flags a patient who, over a few months, has visited multiple specialists for similar issues, but the patient’s medical history shows no prior conditions that would necessitate these visits. The AI also detects that the same patient has been billed for a combination of treatments that do not align with typical medical protocols. Further investigation reveals that this patient has been complicit in a scheme where fraudulent claims are made for unnecessary treatments.
This type of anomaly detection enables hospitals to identify patients who may be involved in fraud, as well as providers who may be overbilling or conducting unnecessary tests.
5. Reduction in Human Error through Continuous Learning
Generative AI continuously learns from new data, which means that its ability to detect fraud improves over time. Unlike traditional rule-based systems that rely on preset criteria, generative AI adapts to new fraud tactics by learning from past cases, improving its accuracy, and minimizing human error.
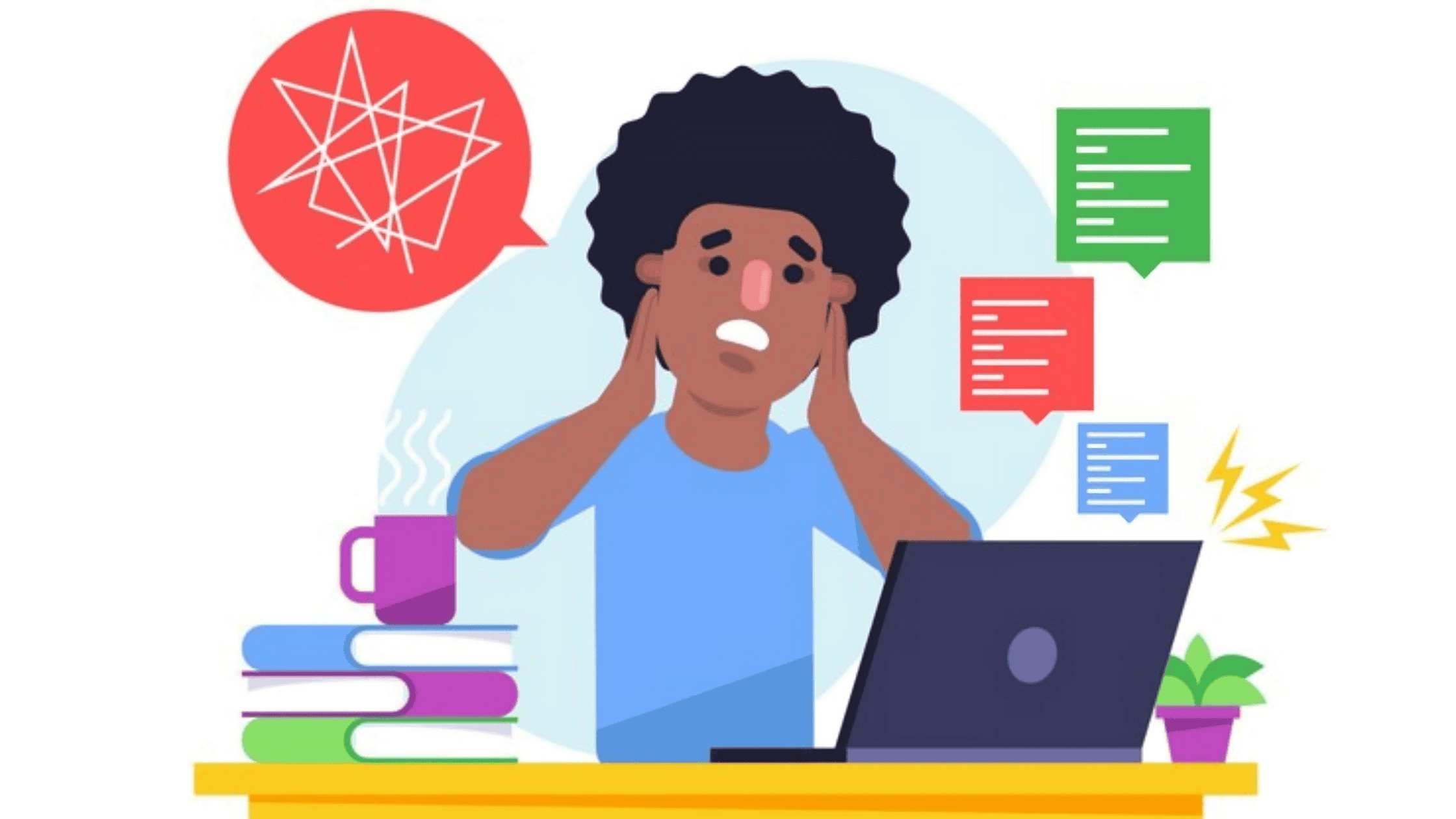
Quick example:
In a large network of clinics, a fraud detection system is set up to review incoming claims. Initially, the system is manually programmed to identify common fraud indicators such as mismatched codes or duplicate claims. However, as fraudsters begin to develop more sophisticated techniques, the system starts missing these new patterns. Enter generative AI.
Over time, the AI is fed with more claims data and learns from patterns that were previously missed. It eventually detects an emerging trend where multiple claims for similar services are submitted at different times across different locations but are the same patient. The AI detects this discrepancy and alerts the fraud team, who can investigate and stop the fraudulent claims from being processed.
Generative AI’s ability to continuously learn and improve reduces the risk of missing new and evolving fraud tactics, enhancing the overall efficiency of fraud prevention efforts.
Prevent healthcare fraud with the leading Gen AI development company in the US and beyond
AlignMinds offers comprehensive AI consulting services, guiding businesses in understanding, planning, and implementing AI-powered fraud detection. We provide AI solutions that are adaptable to diverse business environments, catering to the needs of organizations in the US, Canada, and Australia and beyond. Our commitment is to transform your AI vision into tangible results. From the initial pilot phase to full-scale production, our expertise ensures that AI integration is smooth, efficient, and impactful.
Want to get the most out of Gen AI for fraud prevention? Get in touch now.
Comments
Post a Comment